The Buyers Guides for AI Platforms Classifies and Rates Software Providers
I am happy to share insights gleaned from our latest Buyers Guide, an assessment of how well software providers’ offerings meet buyers’ requirements. The AI Platforms: Ventana Research Buyers Guide is the distillation of a year of market and product research by Ventana Research.
While Artificial intelligence (AI) has evolved over many decades, it is front and center due to a combination of factors that have dramatically increased awareness of and investment in technologies to support its purpose. Since its inception, AI has provided value no matter how or where it has been applied: helping to prevent credit card fraud, segmenting customers for more effective marketing campaigns, making recommendations for the best next action, predicting maintenance routines to prevent machine failures and many other use cases. Recently, the advent of generative AI (GenAI) has brought heightened attention to the AI market. ISG Buyer Behavior research shows that nearly one-half (49%) of enterprise AI budgets are being allocated to GenAI investments. This heightened awareness of AI has brought a focus on the broader issues of developing, deploying and maintaining AI applications in enterprise production environments.
AI Platforms have existed for decades, but several challenges have prevented wide-spread adoption.
Ventana Research defines AI Platforms as those platforms that include the ability to prepare, deploy and maintain AI models. Preparing a model requires accessing and preparing data used in the modeling process. Training a model requires tooling for data scientists to explore, compare and optimize models developed using different algorithms and parameters. Deploying and maintaining models require governance and monitoring frameworks to ensure that models comply with both internal policies as well as regulatory requirements. And, when models are out of compliance, the platforms should provide mechanisms to retrain and redeploy new models that are compliant.
AI Platforms have existed for decades, but several challenges have prevented widespread adoption. Among the challenges were the costs and technical difficulties in collecting and processing the volumes of data needed to produce accurate models. For example, the data from many transactions must be collected and analyzed in order to predict fraudulent transactions. But since the overwhelming majority of transactions are legitimate, many transactions must be analyzed so that the models have enough observations of fraudulent transactions to make accurate predictions. As scale-out computing and object storage have driven down costs, it is now much more economically feasible to collect and process all this data.
Another challenge has been the lack of skills needed to create and deploy AI models. Our research shows that hiring and retaining AI-talented resources is the most challenging technical role to fill and the lack of expertise is the most significant challenge enterprises face in adopting AI. GenAI has brought new attention to the domain of AI and has the promise to make AI much more accessible and more easily utilized in a broader portion of the workforce and the general public. In fact, 85% of enterprises believe that investment in GenAI technology in the next 24 months is important or critical. And the ISG Buyer Market Lens AI research finds enterprises are experiencing positive outcomes from their AI investments. Nearly 9 in 10 (88%) report positive outcomes when using AI for search that proactively answers questions. A similar proportion (87%) report positive outcomes in the interpretation of tabular data
While the rise of GenAI has been meteoric, enterprises still plan to invest one-half of their AI spend on predictive or traditional AI. The most common tasks where GenAI is being applied include natural language processing (NLP) such as chatbots/copilots/assistants, extracting information from and summarizing documents, and assisting with software development tasks such as code generation and application migration. GenAI is expected to have a bigger impact in these areas than predictive AI.
However, in areas such as credit risk, fraud detection, algorithmic trading and even customer acquisition, predictive AI is expected to have a bigger impact. Part of the reason, as noted previously, is that predictive AI is hard. Fine tuning models requires knowledge of not just the algorithms, but also their various parameters and the appropriate data preparation techniques. Data scientists must also understand biases in the data and issues in the training process such as overfitting or poor sampling.
Developing and deploying AI models is a multistep process, beginning with collecting and curating the data that will be used to create the model. Once a model is developed and tuned using the training data, it needs to be tested to determine its accuracy and performance. Then the model needs to be applied in an operational application or process.
For example, in a customer service application, a predictive AI model might make a recommendation for how a representative should respond to the customer’s situation. Similarly, a self-service customer application might use a large language model (LLM) to provide a chatbot or guided experience to deliver those recommendations.
The process does not conclude once a model is deployed. Enterprises need to monitor and maintain the models, ensuring they continue to be accurate and relevant as market conditions change. Realistically, it is only a matter of time before a model’s accuracy has declined to the point where it can be replaced by another, more accurate model. The new model may simply be the result of retraining the old model on new data or it may be the result of using different modeling techniques. In either case, the models must be monitored constantly and updated as and when necessary. In the case of third-party LLMs, the providers are constantly updating and improving their models, so enterprises need to be prepared to deploy the newer models as well.
Data is flowing throughout these processes. Considerable time and effort are invested in preparing data to feed into predictive models. Feature engineering requires exploration and experimentation with the data. Once the features are identified, robust repeatable processes are needed to create data pipelines that feed these features into the models. In the case of GenAI, data—often in the form of documents—feeds custom LLM development or fine tuning. Additional data flows through the prompting process to direct LLMs to provide more specific and more accurate responses. Enterprises must govern these data flows to ensure compliance with internal policies and regulatory requirements. The regulatory environment is emerging and evolving, with the European Union passing the AI Act, the U.S. issuing an Executive Order on responsible development of AI and dozens of U.S. states either enacting or proposing AI regulations.
The processes of moving AI to production, keeping models up to date and including governance throughout are collectively referred to as machine learning operations (MLOps) or, in the case of LLMs, LLMOps. Software providers were slow to recognize that the lack of MLOps/LLMOps tooling was inhibiting successful use of AI. Enterprises were left to their own devices to create scripts and cobble together solutions to address these issues. Fortunately, AI software providers have expanded their platforms to address many of these capabilities, and specialist providers have emerged with a focus on MLOps/LLMOps. In fact, we assert that by 2026, 4 in 5 enterprises will use MLOps and LLMOps tools to improve the quality and governance of their AI/ML efforts.
All these capabilities are important to maximize the success of AI investments. As a result, our evaluation of AI Platform software providers considers each of them in this Buyers Guide. Our framework includes data preparation to ensure quality data is used to train and test models. We also consider the range of modeling algorithms available, as well as the tuning, optimization and testing options supported. Similarly, the range of AutoML functionality is included, along with data scientist tooling to understand how well the platform boosts productivity. Today, no platform would be complete without GenAI. And finally, MLOps/LLMOps must be considered to evaluate how effectively the platform can be used to put models into production and to maintain those models over time.
Technology improvements alone are not enough to improve the use of data in an enterprise.
ISG advises enterprises that a methodical approach is essential to maximize competitiveness. To improve the performance of your enterprise’s people, process, information and technology components, it is critical to select the right provider and product. Many need to improve in this regard. Our research analysis placed fewer than 1 in 5 enterprises (18%) at the highest Innovative level of performance in their use of analytics and data. However, caution is appropriate here: technology improvements alone are not enough to improve the use of data in an enterprise. Doing so requires applying a balanced set of upgrades that also include efforts to improve people skills and processes. The research finds fewer than 1 in 6 enterprises (15%) at the highest Innovative level of performance for process in relation to analytics and data, and fewer than 1 in 8 (12%) at the Innovative level of performance for people.
The overall AI Platforms Buyers Guide is designed to provide a holistic view of a software provider’s ability to serve a combination of traditional AI, GenAI and MLOps/LLMOps workloads with either a single AI platform product or set of AI platform products. As such, the AI Platforms Buyers Guide includes the full breadth of overall AI capabilities. Our evaluation also considered whether the capabilities were available from a software provider in a single offering or a suite of products or cloud services.
The overall AI Platform Buyers Guide includes evaluation of data preparation, types of modeling, AutoML, GenAI, developer and data scientist tooling, MLOps/LLMOps, advanced model optimization and investment. To be included in the Buyers Guide, the software provider must include data preparation, AI/ML modeling, developer and data scientist tooling, model deployment, and model tuning and optimization.
The Buyers Guide for AI Platforms is the result of our evaluation of the following software providers that offer products that address key elements of AI platforms to support a combination of AI, GenAI and MLOps/LLMOps workloads: Alibaba Cloud, Altair, Alteryx, Anaconda, Amazon Web Services (AWS), C3 AI, Cloudera, Databricks, Dataiku, DataRobot, Domino Data Lab, Google, H20.ai, IBM, MathWorks, Microsoft, NVIDIA, Oracle, Palantir, Red Hat, Salesforce, SAP, SAS, Snowflake and Teradata.
This research-based index evaluates the full business and information technology value of AI platforms software offerings. I encourage you to learn more about our Buyers Guide and its effectiveness as a provider selection and RFI/RFP tool.
We urge organizations to do a thorough job of evaluating AI platforms offerings in this Buyers Guide as both the results of our in-depth analysis of these software providers and as an evaluation methodology. The Buyers Guide can be used to evaluate existing suppliers, plus provides evaluation criteria for new projects. Using it can shorten the cycle time for an RFP and the definition of an RFI.
The Buyers Guide for AI Platforms in 2024 finds Oracle first on the list, followed by AWS and IBM.
Software providers that rated in the top three of any category ﹘ including the product and customer experience dimensions ﹘ earn the designation of Leader.
The Leaders in Product Experience are:
- Oracle
- AWS
- IBM
- Dataiku
The Leaders in Customer Experience are:
- Databricks
- Microsoft
- SAP
The Leaders across any of the seven categories are:
- Oracle, which has achieved this rating in five of the seven categories.
- SAP in four categories.
- AWS in three categories.
- Alteryx and Databricks in two categories.
- Dataiku, DataRobot, Google, H2O.ai, Microsoft and Teradata in one category.
The Buyers Guide for GenAI evaluates the software providers that are part of the AI Platform Buyers Guide with specific GenAI support as well as those focused solely on GenAI. The specific GenAI Buyers Guide uses portions of the AI Platform capability framework and includes the evaluation of specific data preparation, development of large language models (LLMs) and foundation model, generative capabilities, developer and data scientist tooling and LLMOps. To be included in the Buyers Guide the software provider must support specific capabilities for data preparation, large language modeling and tuning, GenAI and developer tooling support.
This Buyers Guide research evaluates the following software providers that offer products that address key elements of AI platforms specifically for GenAI: Alibaba Cloud, Altair, Amazon Web Services (AWS), Anthropic, C3 AI, Cohere, Databricks, Dataiku, DataRobot, Domino Data Lab, Google, H20.ai, Hugging Face, IBM, Microsoft, Nvidia, OpenAI, Oracle, Palantir, Salesforce, SAP, Snowflake and Teradata.
The Buyers Guide for GenAI in 2024 finds Oracle first on the list, followed by Databricks and Microsoft.
Software providers that rated in the top three of any category ﹘ including the product and customer experience dimensions ﹘ earn the designation of Leader.
The Leaders in Product Experience are:
- Oracle
- IBM
The Leaders in Customer Experience are:
- Databricks
- Microsoft
- SAP
The Leaders across any of the seven categories are:
- Oracle, which has achieved this rating in five of the seven categories.
- SAP in four categories.
- Databricks and AWS in three categories.
- Google in two categories.
- Hugging Face, IBM, Microsoft, Teradata and Salesforce in one category.
For software providers that are part of the MLOps Buyers Guide, only those with specific MLOps support were considered for inclusion in the evaluation. The specific MLOps Buyers Guide uses portions the AI Platform capability framework and includes the evaluation of specific AI/ML modeling, developer and data scientist tooling, MLOps and advanced model optimization. To be included in the Buyers Guide the software provider must include specific capabilities for deployment, monitoring, and governance of models and developer tooling.
This Buyers Guide research evaluates the following software providers that offer products that address key elements of MLOps: Alibaba Cloud, Altair, Alteryx, Amazon Web Services (AWS), Anaconda, C3 AI, Cloudera, Databricks, Dataiku, DataRobot, Domino Data Lab, Google, H20.ai, IBM, Microsoft, Nvidia, Oracle, Palantir, Red Hat, SAP, SAS, Snowflake and Teradata.
The Buyers Guide for AI Platforms in 2024 finds Oracle first on the list, followed by AWS and Databricks.
Software providers that rated in the top three of any category ﹘ including the product and customer experience dimensions ﹘ earn the designation of Leader.
The Leaders in Product Experience are:
- Oracle
- AWS
- Dataiku
The Leaders in Customer Experience are:
- Databricks
- Microsoft
- SAP
The Leaders across any of the seven categories are:
- Oracle, which has achieved this rating in five of the seven categories.
- SAP and AWS in four categories.
- Alteryx and Databricks in two categories.
- Dataiku, DataRobot, Google, Microsoft and Teradata in one category.
The overall performance chart provides a visual representation of how providers rate across product and customer experience. Software providers with products scoring higher in a weighted rating of the five product experience categories place farther to the right. The combination of ratings for the two customer experience categories determines their placement on the vertical axis. As a result, providers that place closer to the upper-right are “exemplary” and rated higher than those closer to the lower-left and identified as providers of “merit.” Software providers that excelled at customer experience over product experience have an “assurance” rating, and those excelling instead in product experience have an “innovative” rating.
Note that close provider scores should not be taken to imply that the packages evaluated are functionally identical or equally well-suited for use by every enterprise or process. Although there is a high degree of commonality in how organizations handle AI Platforms, there are many idiosyncrasies and differences that can make one provider’s offering a better fit than another.
Our firm has made every effort to encompass in this Buyers Guide the overall product and customer experience from our AI Platforms blueprint, which we believe reflects what a well-crafted RFP should contain. Even so, there may be additional areas that affect which software provider and products best fit an enterprise’s particular requirements. Therefore, while this research is complete as it stands, utilizing it in your own organizational context is critical to ensure that products deliver the highest level of support for your projects.You can find more details on our community as well as on our expertise in the research for these Buyers Guides:
Regards,
David Menninger
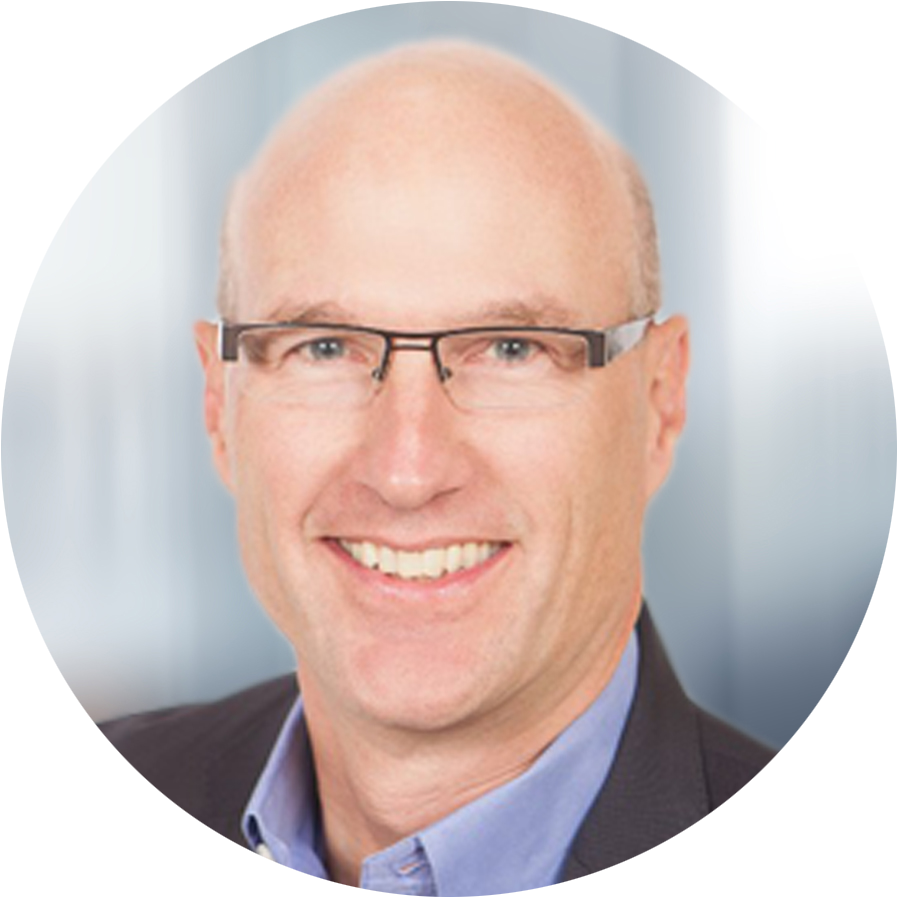
David Menninger
Executive Director, Technology Research
David Menninger leads technology software research and advisory for Ventana Research, now part of ISG. Building on over three decades of enterprise software leadership experience, he guides the team responsible for a wide range of technology-focused data and analytics topics, including AI for IT and AI-infused software.